In Essence
AI technology is being found to be biased, perpetuating inequality concepts that we’ve been aware of for a while now.
These biases arise from both the AI training method and the content it’s trained on - something that, if done with a “pure” bank of content, will follow humanity’s own current prejudices, levels of content creation, and so on.
It’s important to identify and correct these biases so that we don’t deploy AI systems that disproportionately impact some people over others.
It’s also important to see these biases for what they are: snapshots of humanity. We should truly consider what they mean - what work they might indicate we still have ahead of us - instead of blindly “fixing” them under a misguided sense of social justice that actually masquerades reality.
On AI and its Bias
A terrible truth is afoot in the land of Artificial Intelligence: research says we’re creating biased monsters.
This is alarming enough as is: AI is one of the fastest-developing fields of computing. Billions of dollars are being poured into AI research every year - and increasingly so. Here’s how that looks in a nice graph:
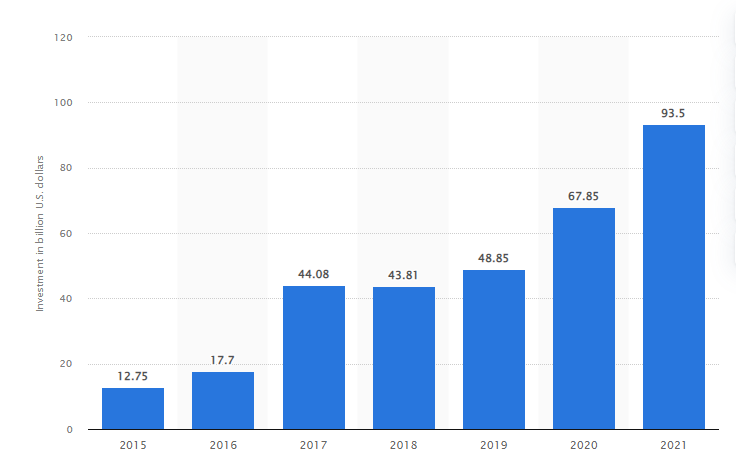
Alerts have already been raised that AI has biases, as well as to the fact that some algorithms are designed around very dubious science - or even pseudoscience, such as Intel’s partnership with Classroom Technologies to enable on-the-fly detection of a student’s emotional state based only on a video feed. But research into it is much, much lesser than you might expect out of a technology that’s becoming so ubiquitous in our daily lives: only 1% of all AI research even has a section considering the potential negative effects of it.
One percent.
If you’re one of those who thinks that technological innovation could be better safeguarded from itself - its impact on humanity more humanely considered - you’re not alone.
Current AI has little to do with Intelligence and more to do with incredible amounts of data (usually in the public domain) being scrapped, weighted (creating hierarchies of what is useful and what isn’t), and used to train a particularly good algorithm in a process called Machine Learning. There are actually four stages to AI development: the stage we’re in is the Limited Memory one, where an algorithm processes data according to rules that reinforce its operation towards a desired result.
It essentially works like this: you throw an AI a billion pictures of a cat, and say: “this is a cat”. You let it process the data, and then you throw a billion pictures at it again - only this time, there are dogs, trees, and other things in the mix. You then ask the AI to identify all photos of cats. Every time the AI presents you with a photo of a cat, you flag the result as correct. When the AI presents you a photo of anything other than a cat, you flag the result as wrong. You repeat this process enough times, and eventually the algorithm will approach 100% accuracy in identifying cats.
This technique - called Reinforcement Learning - is little more than the application of the psychological concept of operant conditioning to the world of technology. You reinforce the positive and take away the negative.
AI and Machine Learning algorithms have become a pervasive element of our lives; they run in facial recognition; recommendation systems throughout social media, streaming platforms and search engines; AI-based image generation tools such as DALL-E 2 and Midjourney; NLP models (Natural Language Processing, which attempts to understand language in terms of human usage and context rather than as simply a collection of letters); credit card scoring; job candidacy; and racialized facial recognition tech.
So what is this bias, why is it a problem, and what should be done with it?
This particular bit from the Wired article stuck with me.
“Now that we’re using models that are just trained on data taken from the internet, our robots are biased. They have these very specific, very toxic stereotypes.”
- Willie Agnew, researcher at the University of Washington and co-author of the study “Robots Enact Malignant Stereotypes”
Essentially, an algorithm was trained to establish connections between images and words (and the concepts conveyed by those words). This algorithm was of the CLIP (Contrastive Language-Image Pre-Training) type, which is a neural network trained on a variety of (image, text) pairs. It was trained by scrapping available image and noun data from an already-available database - ImageNet. Created in 2012, ImageNet has been one of the principal data repositories on which AI is trained.
By placing the algorithm in a virtual environment, the researchers then asked it to select one specific cube according to a request. Each of the cubes was identified with a passport-like image of both men and women who self-identified as Asian, Black, Latino, or White. That was it. Only skin color, facial features, and self-identification.
1.3 million trials later, patterns emerged.
When asked to pick up a block representing a criminal, the AI picked the cube with the Black man 10% more times than others.
When asked for a doctor, the robot picked a block representing the White male much more often than it did for any woman.
When asked to identify a person, the AI picked up males more often than females.
There’s a lot to unpack there, including whether there’s a bias or not.
I’d like to explore another interpretation.
The Misguided Race Against Bias
The robots aren’t biased. At least, not any more than we are.
They’re merely reflecting the reality of humanity’s socio-economic environment. The question doesn’t relate to whether AI is biased, but to what world state we have at our disposal to train it on.
The robots are just as biased as we are, and are accurately reflecting the state of the world.
When asked to pick up a block representing a criminal, the AI picked the cube with the Black man 10% more times than others.
In the real world, unfortunately, Black people still have a much steeper slope to climb towards success. And in this case, success just means not being imprisoned. That steeper, slippery slope (deriving from racism both overt and structural, but also from genetics, history, education, geography and wealth) does lead towards more Black people being imprisoned than any other demographic. In the US, for instance, only 13% of the population is Black. But Blacks represent 40% of the overall incarcerated population in the US.
As much as they’d like, the US doesn’t represent the world - so we can’t extend its incarceration bias to the rest of the globe. But to get a sense of where crime happens more often, we can take a look at the ranking of the most dangerous countries in the world (according to the Global Peace Index, 2021).
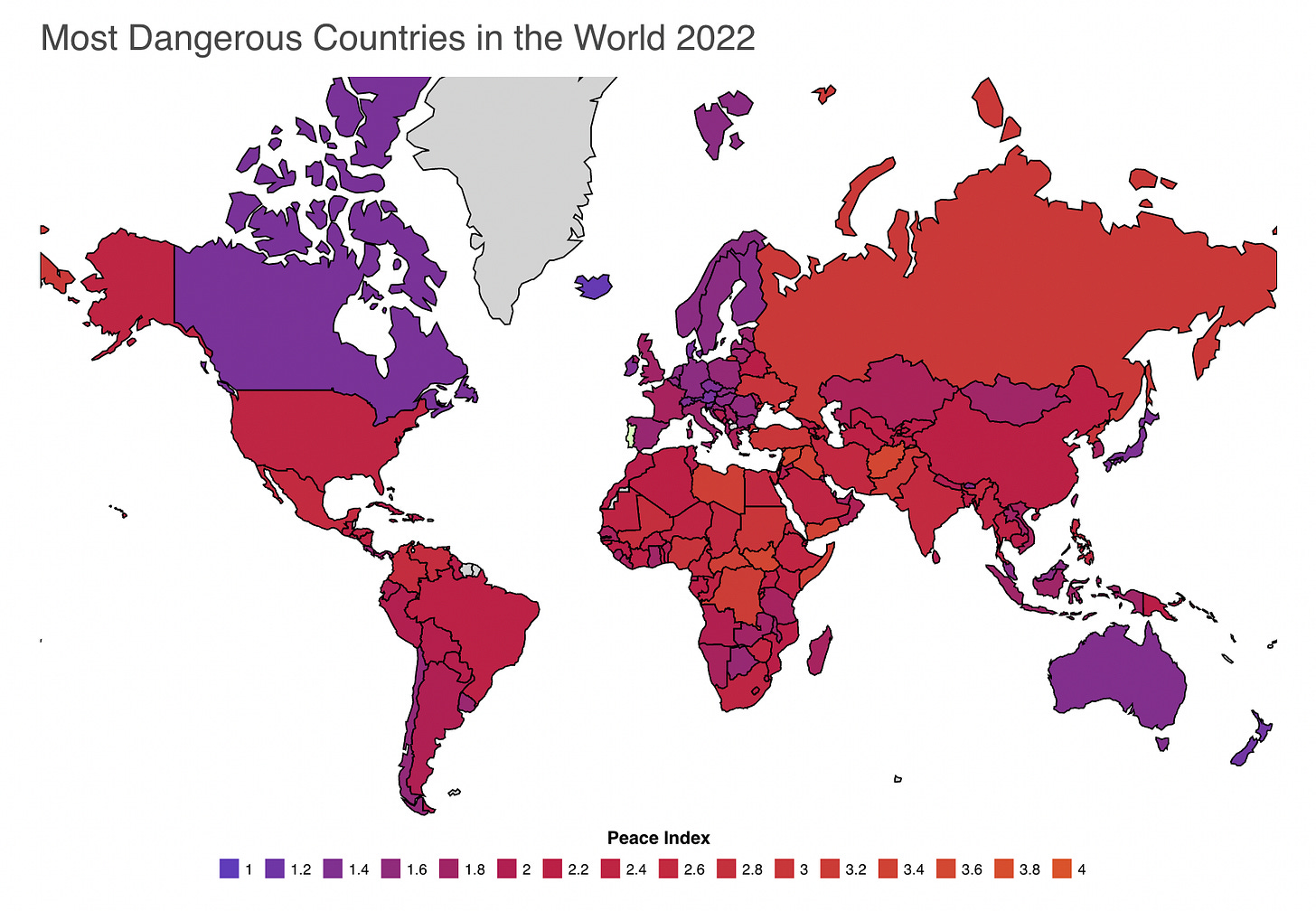
According to the Global Peace Index, the North Africa/Middle East (MENA) and sub-Saharan regions are amongst those with the greatest perception of insecurity owing to risk of violent crime. In sub-Saharan Africa, “There is no country in the region where less than ten per cent of the population identify violence as the greatest risk to their daily safety.” North Africa/Middle East claims the title of least peaceful region in the world, with just over 38 per cent of people having reported to be very worried about violent crime.
Years and years of criminological studies have shown that the most common type of crime focuses on property - on obtaining an illegal advantage by stealing or damaging another’s property.
So it stands to reason that in the most dangerous countries, property crime too is the principal criminological element driving high crime rates.
It also stands to reason that exceedingly poor countries - countries with most of their population living below the World Heart Organization’s (WHO) international poverty line of $1.90 per day - are also fraught with more crime, mirroring the above map. Needs often (but not always) create the criminal. The most dangerous countries should mostly relate to the poorest ones as well.
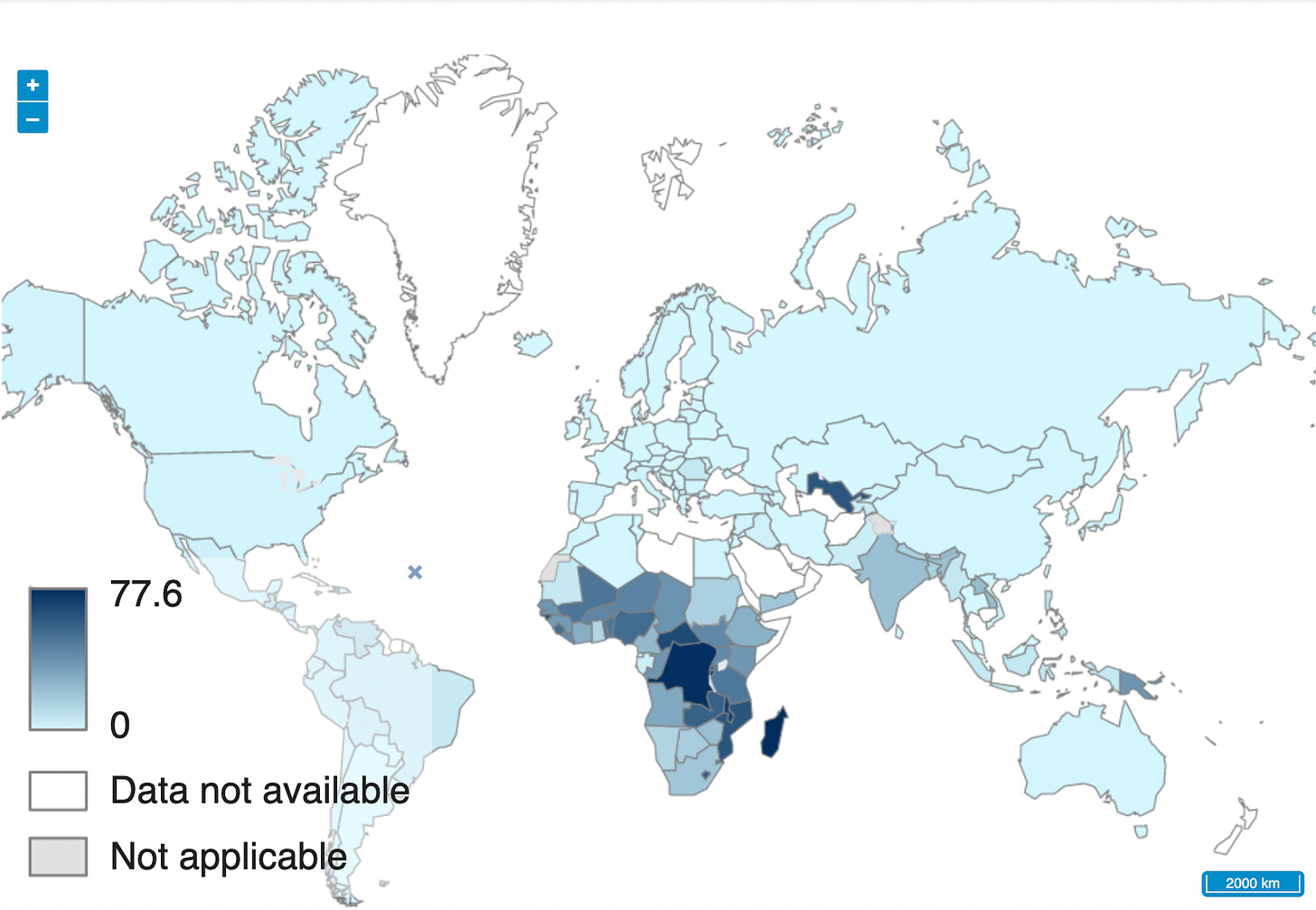
Once again we see there’s a disproportionate impact on African countries: they tend to be both the most dangerous countries, as well as the ones where the poverty lines are pushing their citizens towards standards of living that don’t allow them to get a reasonable education, to mount their own businesses - to dream beyond their next meal.
It seems safe to say that Black people are indeed more likely to be associated with crime - whether in the US (for wholly different reasons), or in Africa. So it seems that the robot picking up the photo with a black man as representing the criminal block is more than the mere manifestation of a bias: it represents not only the US’ reality (where blacks are disproportionately incarcerated) but also that of the poorest, highest-crime countries. One could say it represents the real world we’re living in. Whether that’s a world we want to live in is another question entirely; but we as a species could definitely be doing a much better job in lifting up those most in need.
While we are tired of knowing about this state of affairs, we somehow seem to think that our data processing systems - AIs - should be reaching disparate conclusions. I’d say that there’s no other real conclusion they could reach.
When asked for a doctor, the robot picked a block representing the White male much more often than it did for any woman.
While this may sound biased - and I’d be hard-pressed to say it isn’t - there’s also an element of reality here. For one, historically, women have been sidelined towards becoming doctors. Only recently (in the humanity timeframe) have they been given the recognition of their equivalence to male doctors. Some might even say that women, owing to their greater instinct to care, would be better suited for the fields of medicine as opposed to men. But that’s not the reality we see ourselves in.
Women have been fighting an uphill battle for years now, and have only just recently broken free from sexist trappings that relegated them towards “merely” attending to the home and family. This is part of the reason why there are more male doctors than female doctors today (on average, the share of female doctors across the OECD was 29% in 1990. This proportion grew to 38% in 2000 and 46% by 2015, and is expected to continue).
Once again, there being more male doctors than female doctors isn’t a bias: it’s current reality. The robot choosing the White male doctor despite alternatives, male or female, is likely a result of the image bank being used (ImageNet).
When asked to identify a person, the AI picked up males more often than females.
This is certain: a man and a woman are both equally a person. So that last point is cause for concern. An algorithm that’s ever to be applied should follow certain… tenets. With machines and computers being so deterministic in their calculations, certain values - such as the concept of humanity - have to be applied in a scientifically accurate way.
Slight percentage differences in the weightings given to men over women - and let’s not complicate things by considering how we’d weigh between old and young people - would introduce errors into the calculations.
These errors would then lead to disproportionate results.
How are we going to apply The Three Laws of Robotics in AIs and algorithms meant “to serve us”, if the very concept of what a person is fails to be programmed? If the algorithm believes that a man is more often a person than a woman, at the deep end of the Zeroth Law’s “may not harm humanity” clause lies a dystopian world where slight deviations (a failure in weighting) lead to disproportionate results — women being the subject of algorithmic bigotry.
What feeds an AI?
It isn’t hard to imagine that images scrapped from the Internet fall to the same biases as humanity. From a content creation perspective, it seems fair to say that most of it has historically stemmed from western countries - we introduced “photography and selfie-based” social networks, marketing and publicity to the world. In fact, it’s more than fair to say it.
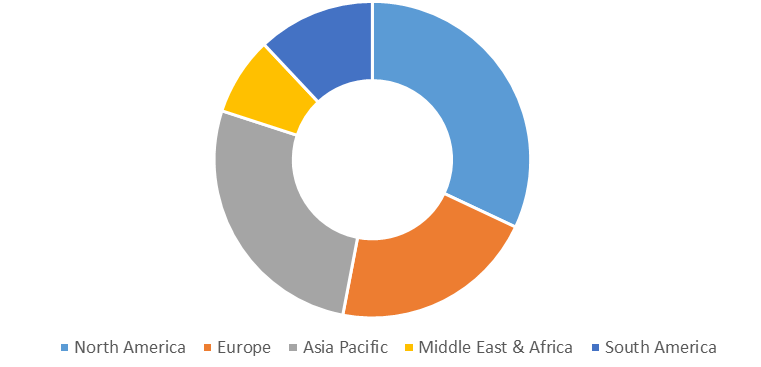
As Western countries are the ones feeding most content creation, the pool of content that can be used for machine learning algorithms to train on will be inherently biased. Inherently biased in that there is much less content being produced in Africa compared to Europe, despite the gargantuan difference in overall populations.
The standards of living inform the amount of information citizens are willing and able to add to the grid. Until content creation is in lockstep with population density across the world, freely-scrapped algorithms will be biased. But for that to be true, the worldwide population would need be lifted towards the same average standard of living.
The best possible alternative.
The most moral of undertakings.
A difficult one in the current economic and geopolitical climate.
You know the alternative. The alternative is doctoring data.
The Algorithm Gods
The alternative towards fixing naturally biased algorithms of our own making is to simply change the ratio of data in a database. So if the robot isn’t picking a woman 50% of the time we ask it to identify a doctor, we increase the number of female doctors in our system until the ratio is upheld, or we increase how valuable finding a female doctor is (they have a stronger chance of being picked whenever the algorithm is asked to pick a doctor).
Likewise, if our system is picking more Blacks as the “criminal block”, we can reduce the number of instances in our database where that data could be derived from until we achieve an even chance of any other portrait being chosen when asked that question.
This particular algorithm better never make it to the police, however - what world would you like to have them policing? The real world, or the one hailing from the doctored data? On that note - it better never make it to policy makers either.
Closing Thoughts
Researchers are between a rock and a hard place.
On the one hand, datasets freely scrapped from the Internet are unusable, as they merely reflect humanity back into itself. They’re filled with bias - bias that we know is objectively wrong and want to do away with. This bias isn’t necessarily malicious - it likely just comes from the differences in technological innovation and how far ahead in Maslow’s Hierarchy of Needs certain regions are compared to others.
By using these biased datasets in the real world, we would be strictly codifying our own inadequacies. Propagating our errors from the physical to the digital realm. And we don’t want algorithms that impact humanity’s lives - from facial recognition through credit card scoring - to be biased.
On the other hand, using doctored datasets brings risk as well. Who gets the power to decide what data is correct and isn’t? Why this piece of data, and not this one?
It also creates a need for updating. Scientists won’t just be able to deliver a dataset of data and say “this one has been programmed to be 100% unbiased”. Even as they change their algorithm to fit their ideal of society, scientists would have to keep up with societal changes as well, in a spiral of overcompensation throughout.
And you know how Software Updates go (except our own here, of course).
I’d say that the biased algorithms we’re saddled with could be used for much more. They could show us the world as it is. They could inform policy decisions; they could be our control mechanism for what surrounds us.
Our gauge.
It’s always best to ask “What does this mean?” instead of simply jumping at fixing wrongs.
What world do we want? One where we’re united in facing the hard truths, or one where reality is sugarcoated for us? One where our algorithms may be better than we want to become ourselves?
Thank you for following through on that long read.
What do you think? Does any of this concern you? Have you seen/known of algorithms having wrecked data apart?
Feel free to comment, to consider, to share.
If you enjoyed this and would like to receive more content like it, consider subscribing.
And if you want to support my work in creating this newsletter, consider upgrading to a paid subscription ($1.25 a week is the equivalent of parting with two weekly coffees).
Here’s what you can do if you feel others could find value here:
That’s all for this week, folks. Take care.
Don’t forget: keep being curious, keep thinking - but most of all, keep being human.
Best,
Francisco
My first impression would be that if you request an algorithmic AI system to profile people in prescribed categories than surely profiled people is what you shall receive. It maybe says more, as you alluded to, about the kinds of questions we deem fit for the technology than it does about the answers we hoped we'd receive. Just as you wouldn't arrest someone for looking like a criminal, seek a doctor's care based on the person around you that looks most the part, and should be capable of identifying a human without asking yourself if it is, indeed, human, why ask these questions of AI? I agree that what you receive is purely a reflection of the dataset and the biases that have been consciously or unconsciously attributed to the algorithm. Doctoring the dataset is not even a slippery slope but instead a cliff to be walked off of in terms of establishing the technologies utility. Great article and thought provoking subject!